Predictive learning analytics: An empowering tool in the hands of online teachers
April 29, 2021
Keywords: learning analytics, Online Teaching, Predictive Analytics
Target readers: researchers, leaders, educators
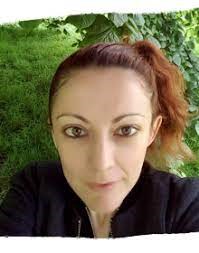
Author: Christothea Herodotou
Christothea Herodotou is an Associate Professor at the Open University UK with research interests in predictive learning analytics, citizen science, and mobile learning. She led the evaluation of the Early Alert Indicators project that enabled the adoption and use of predictive learning analytics across the Open University.
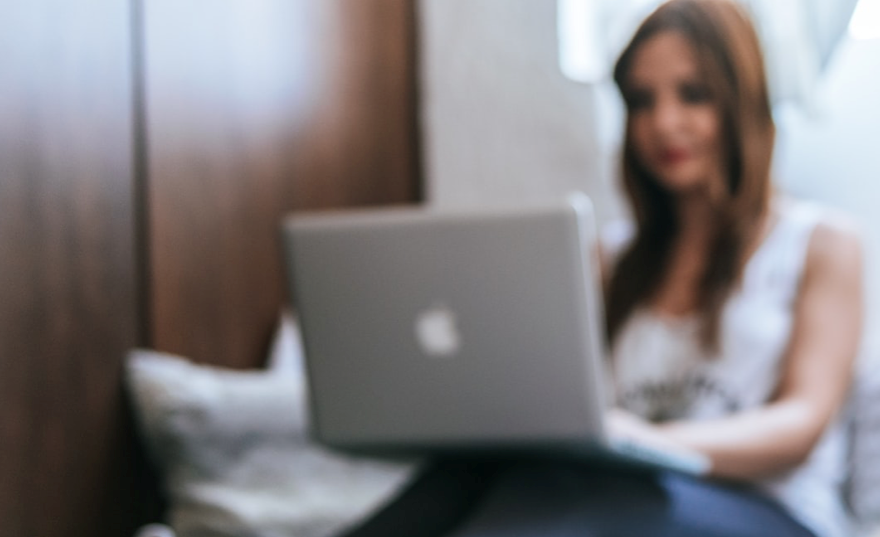
Photo by Bruno Emmanuelle on Unsplash
Predictive learning analytics: An empowering tool in the hands of online teachers
In this blog, you will learn Emma’s story. Emma is an imaginary name, yet her story as a student is a real one. Emma’s story was tracked because of a proactive teacher who was regularly checking on an intelligent tool that predicted students at risk of failing.
Emma is an engineering student with a black and minority ethnic background. This is her first time at a university, studying from a distance. She is doing really well with her studies - in her first assignment she got 100% and 86% in her second. She is now expecting a baby. Yet, she hasn’t informed the university. She is concerned that she won’t be allowed to carry on with her studies. Her performance starts deteriorating and faces difficulties to prepare and submit her third assignment. Will Emma manage to finish this course and her program of studies, or will she quit? Is her dream of becoming an engineer ending here?
What students do when studying is a “black box” in online teaching and learning. In contrast to traditional teaching, online teachers have limited interactions with students and a limited understanding of whether and how students are progressing with their studies. Their role is to design teaching materials, mark and assign grades, take part in forum discussions, and hold online tutorials with students. How could an online teacher know what Emma is going through and potentially support her?
A tool that is intelligent enough to predict whether a student is going to fail could be what Emma’s teacher needs. Such a tool is using predictive learning analytics to forecast or predict early on and before failure, whether a student is struggling with their studies – for example, s/he is not engaging with material online - and may need help. It is a tool that can empower online teachers and complement their teaching practices. It can be another pair of eyes for online teachers who rely on ‘clues’ of what students are doing, as well as for teachers who are teaching to a large number of students (e.g., on campus).
Predictions about the future performance of students are calculated drawing from past data about a student such as their previous performance, socioeconomic status, gender, whether they are new to studying, and their activity online such as how frequently they check on material hosted on a virtual learning environment.
These predictions are then presented in a graph or a table, in a dashboard that can be accessed by teachers. Such a graph or a table uses a colour-coded scheme to indicate in green students who are likely to submit their next assignment, in amber those with an average chance of submitting, and in red those who are at risk of failing or not submitting their next assignment.
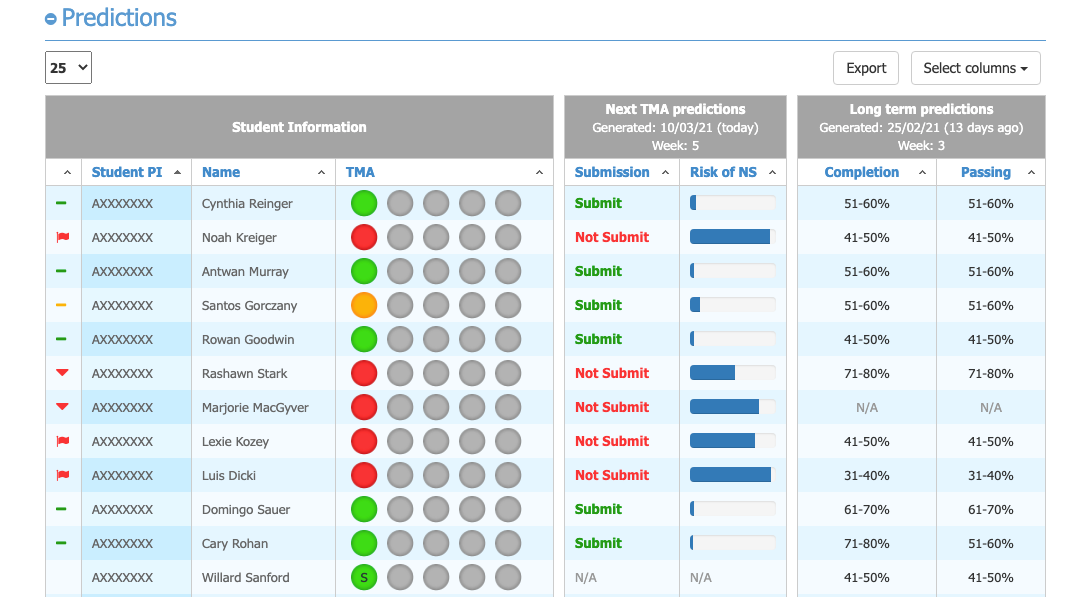
Example screenshot of the Early Alert Indicators dashboard showing (a) short term predictions i.e., the probability a student will submit their next assignment (see TMA) and (b) long term predictions i.e. the probability of completing and passing the course (Note: student names are not real)
These predictions are calculated and updated every week, considering new data about a given student. For example, submitting and passing an assignment can inform predictions of upcoming assignments. Also, it gives information (a percentage) as to whether the student is likely to complete and pass the course as a whole.
Predictions may not always be accurate. For example, they cannot capture personal issues a student like Emma may be facing. The role of the teacher is therefore significant; it is up to the teacher to assess the validity of predictions and decide whether there is a need to get in touch with a student shown as at risk of failing, or not.
It may be the case the teacher is already aware of Emma’s situation and took action to help her (e.g., Emma got in touch with her teacher), or it may be the case this is a new insight the teacher should act upon and explore further with the student.
A student may or may not have the courage to get in touch with their teacher and discuss a difficulty they are facing. Therefore, it becomes crucial for a teacher to be proactive, check on their students and their engagement often, and get in touch when they have insights that they are not performing well.
My team evaluated teachers using predictive learning analytics over a four-year period and found several benefits in using analytics in teaching. Our evaluation demonstrated that teachers who are checking on predictions regularly have students who are performing better.
Two factors predicted future student performance: best previous grade and the use of predictive learning analytics by teachers. Students performed better the year their teachers had access to predictions than the year before, when teachers were using their own ways of monitoring students.
Teachers felt empowered and “on top of their students”. They became more proactive in terms of checking on students regularly and providing support in a timely manner and before a student fails. They saw predictive analytics as improving their teaching practices.
A recent study yet to be published revealed that predictive analytics are of particular help to students who are disadvantaged (coming from a low socio-economic status and a black and minority background) as, when teachers act on predictions and provide support, they can help these students to succeed.
Let’s not forget about Emma…
Her teacher was very keen in using predictive analytics so she saw early on that Emma’s performance was deteriorating and she proactively gave her a call. She discussed her concerns and explained to her that she as a teacher and the university could support her to submit her next assignment, which Emma did and completed with a very good mark. When other personal issues came up after Emma gave birth, predictions helped the teacher to pick on that and give Emma an assignment extension. Emma completed her engineering course with 80% and she is now on track of completing her engineering degree.
To learn more…
Herodotou, C., Rienties, B., Hlosta, M., Boroowa, A., Mangafa, C., & Zdrahal, Z. (2020). The scalable implementation of predictive learning analytics at a distance learning university: Insights from a longitudinal case study. The Internet and Higher Education, 45, 100725.
Herodotou, C., Hlosta, M., Boroowa, A., Rienties, B., Zdrahal, Z., & Mangafa, C. (2019). Empowering online teachers through predictive learning analytics. British Journal of Educational Technology, 50(6), 3064-3079.
Herodotou, C., Rienties, B., Boroowa, A., Zdrahal, Z., & Hlosta, M. (2019). A large-scale implementation of predictive learning analytics in higher education: the teachers’ role and perspective. Educational Technology Research and Development, 67(5), 1273-1306 [OPEN ACCESS]
Herodotou, C., Naydenova, G., Boroowa, A., Gilmour, A., & Rienties, B. (2020). How Can Predictive Learning Analytics and Motivational Interventions Increase Student Retention and Enhance Administrative Support in Distance Education?. Journal of Learning Analytics, 7(2), 72-83. https://doi.org/10.18608/jla.2020.72.4 [OPEN ACCESS]
Hlosta, M., Papathoma, T., & Herodotou, C. (2020, July). Explaining Errors in Predictions of At-Risk Students in Distance Learning Education. In International Conference on Artificial Intelligence in Education (pp. 119-123). Springer, Cham.
Herodotou, C., Rienties, B., Verdin, B., & Boroowa, A. (2019). Predictive learning analytics ‘at scale’: Guidelines to successful implementation in Higher Education based on the case of the Open University UK. Journal of Learning Analytics, 6(1), 85-95. [OPEN ACCESS]